DAQ
Supremacy Technology
The Esculap ESA Project is a multi-faceted initiative blending cutting-edge technologies to advance healthcare applications, with a focus on Distributed Ledger Technology (DLT), Artificial Intelligence (AI), and Quantum Technology (QT). This project, particularly designed for the healthcare sector, utilizes these advanced technologies to enhance various therapeutic processes.
Distributed Ledger Technology (DLT), Artificial Intelligence (AI), and Quantum Technology (QT) fusion is reshaping the digital landscape. Join us on the forefront of this transformative journey for a smarter, faster, and more connected tomorrow!” 🌟 #DAQSupremacyTechnology #InnovationRedefined #EmpoweringTheFuture
One core aspect of Esculap ESA involves the Esculap Generator, a software that uses quantum random number generators and wavelets to modulate signals in physical therapy devices. These signals are applied in therapies that utilize 3D health models for personalized treatment approaches.
The project also integrates cryptocurrency and DLT, offering a digital currency known as ESA Esculap, which serves as a means of exchange within its ecosystem. This currency underpins a variety of healthcare and wellness applications, including AI-driven health history analysis, NFT holograms for medical records, and programmable therapeutic devices​.
Additionally, the Esculap ESA project focuses on fostering a decentralized healthcare ecosystem, promoting investments in DARQ technologies (DLT, AI, HR, QT) to enable seamless interactions and transactions in health-related digital platforms.
Quantum computer clusters with artificial intelligence technology, supremacy.
Quantum computing is the study of how to harness the unique properties of quantum mechanics to solve certain types of problems far faster than on traditional computers. Quantum computers use quantum bits or qubits, which can exist in superpositions of two states, such as 0 and 1, at the same time. This allows them to perform multiple calculations simultaneously, using quantum algorithms and protocols. Quantum computers have the potential to revolutionize various fields, such as cryptography, optimization, simulation, machine learning, and more. [1]
Artificial intelligence is the study of how to create machines or systems that can perform tasks that normally require human intelligence, such as reasoning, learning, decision making, perception, and natural language processing. Artificial intelligence uses various techniques and methods, such as neural networks, deep learning, reinforcement learning, natural language processing, computer vision, and more. Artificial intelligence has the potential to transform various fields, such as healthcare, education, entertainment, security, and more. [2]
Quantum computer clusters with artificial intelligence technology are systems that combine the power and speed of quantum computing with the intelligence and flexibility of artificial intelligence. These systems could enable new capabilities and applications that are beyond the reach of classical computers or artificial intelligence alone. For example, quantum computer clusters with artificial intelligence technology could:
• Solve complex optimization problems, such as scheduling, routing, resource allocation, and more, using quantum annealing or quantum approximate optimization algorithms. [3]
• Simulate complex physical, chemical, or biological systems, such as molecules, materials, or cells, using quantum variational methods or quantum machine learning. [4]
• Enhance machine learning models, such as neural networks, by using quantum data, quantum features, quantum kernels, or quantum circuits.
• Generate novel and creative content, such as images, music, text, or code, using quantum generative models or quantum generative adversarial networks.
Quantum supremacy is a term that refers to the ability of a quantum computer to perform a task that is impossible or intractable for a classical computer. Quantum supremacy is a milestone that demonstrates the advantage and potential of quantum computing over classical computing. Quantum supremacy has been claimed by several groups and organizations, such as Google, IBM, Alibaba, and USTC, using different criteria and benchmarks, such as random quantum circuits, boson sampling, or Gaussian boson sampling. However, quantum supremacy is still a controversial and debated concept, as there are many challenges and limitations that need to be overcome, such as noise, errors, scalability, verification, and usefulness.
Quantum computer clusters with artificial intelligence technology could achieve quantum supremacy in various domains and tasks, such as optimization, simulation, machine learning, and generative AI. However, quantum supremacy is not the ultimate goal of quantum computing or artificial intelligence, but rather a stepping stone towards more practical and impactful applications and innovations. Quantum computer clusters with artificial intelligence technology could also face some ethical and social issues, such as safety, security, privacy, fairness, and responsibility, that need to be addressed and regulated.
[1] https://link.springer.com/article/10.1007/s11082-023-04914-6Â
 [2] https://www.nasa.gov/technology/computing/google-and-nasa-achieve-quantum-supremacy/
 [4] https://spectrum.ieee.org/quantum-memristor
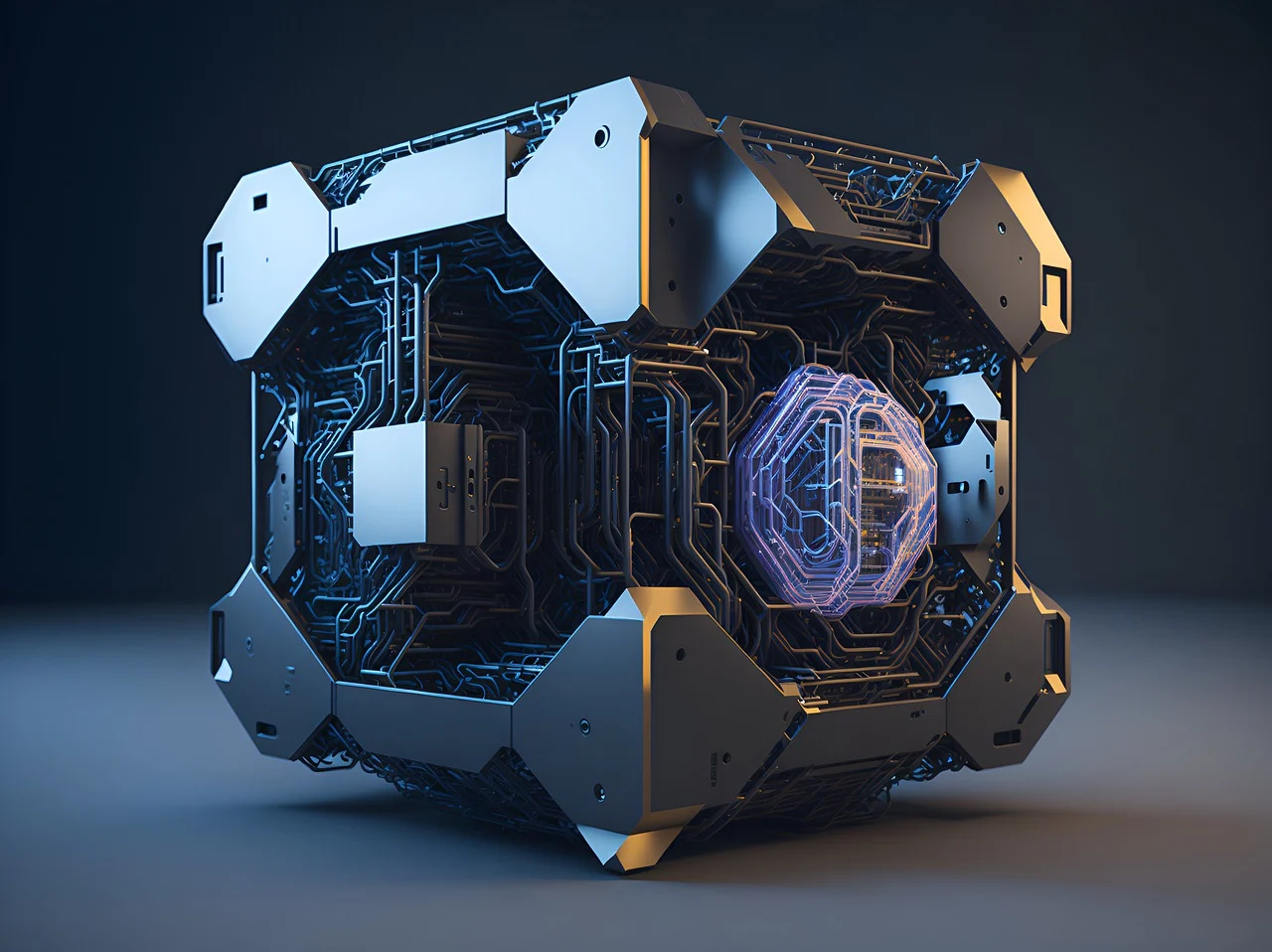
Quantum Computer (artistic representation)Â
https://thefuturisticminds.com/when-will-quantum-computers-be-available/
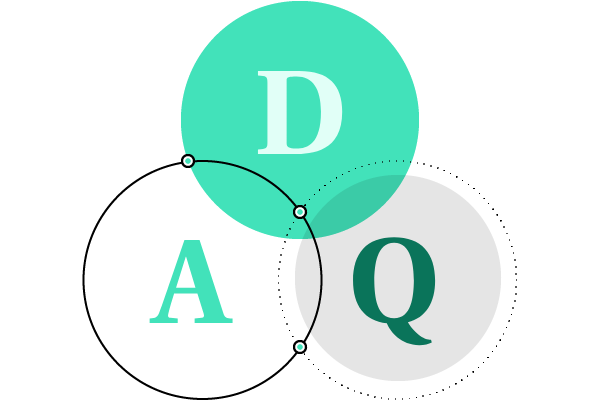
DAQ ~ DLT AI & QT
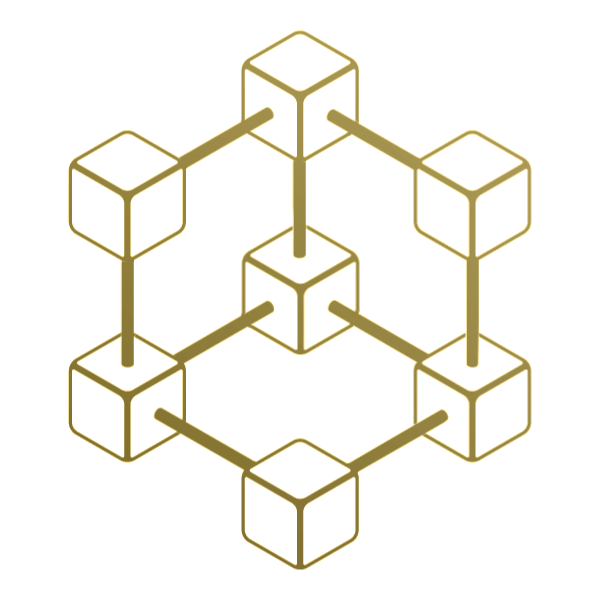
DAQ DLT ~ Distributed Ledger Terchnology
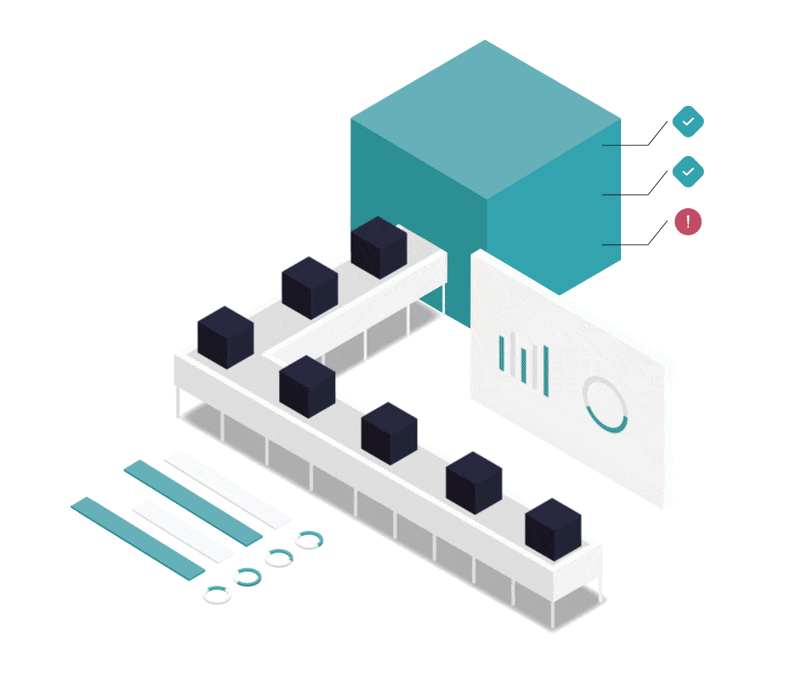
DAQ AI ~ Artificial Intelligence
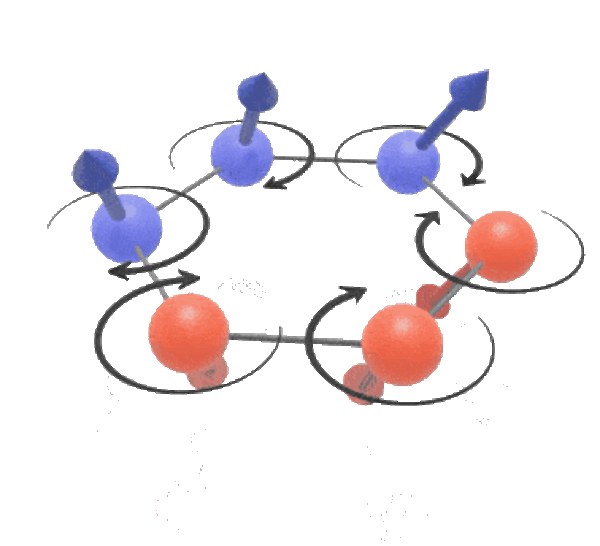
DAQ QT ~ Quantum Technology
DAQ ~ DLT, AI & QT
 DAQ acronym abbreviates DLT (Distributed Ledger Technology), AI (Artificial Intelligence), and QT (Quantum Technology) are distinct yet interrelated technologies that, when converged effectively, can potentially lead to the supremacy of Data Acquisition (DAQ).
DLT (Distributed Ledger Technology): DLT refers to a decentralized database that records transactions across multiple locations in a secure and transparent manner. It ensures that data is immutable, transparent, and tamper-proof. Blockchain, a type of DLT, gained fame through its association with cryptocurrencies like Bitcoin. Its decentralized nature and cryptographic security ensure data integrity and transparency, crucial for acquiring reliable data.
AI (Artificial Intelligence):Technology AI involves the creation of intelligent machines that can mimic cognitive functions such as learning, problem-solving, and decision-making. AI algorithms analyze vast amounts of data to derive insights, make predictions, and automate tasks. When integrated with DLT, AI can enhance the verification, analysis, and interpretation of data stored on distributed ledgers, enabling more accurate and valuable insights.
QT (Quantum Technology): Quantum technology, especially quantum computing, harnesses the principles of quantum mechanics to perform computations that surpass the capabilities of classical computers. Quantum computers have immense processing power and can solve complex problems exponentially faster than traditional computers. When applied to data processing, quantum technology can significantly speed up AI algorithms, enabling faster analysis and handling of vast datasets stored on DLT.
Convergence towards DAQ Supremacy Technology:
-
Enhanced Data Security: DLT ensures data integrity, while quantum-resistant cryptography can fortify DLT against potential threats from quantum computers, securing data for AI-driven analysis.
-
Optimized Data Processing: Quantum computing’s computational prowess can accelerate AI algorithms, enabling faster and more complex data analysis, pattern recognition, and decision-making. This convergence can revolutionize how we process and utilize data.
-
Improved Insights and Decision-Making: The amalgamation of AI’s analytical capabilities with quantum-enhanced speed and DLT’s robustness can yield more accurate, real-time insights from a vast amount of distributed data. This can empower businesses and industries to make data-driven decisions swiftly and accurately.
-
Innovative Applications: The synergy of these technologies can foster innovations across various sectors, including finance, healthcare, logistics, and more. For instance, in healthcare, this convergence can lead to advancements in personalized medicine through better analysis of genomic data.
In summary, the convergence of DLT, AI, and QT creates a powerful ecosystem for acquiring, processing, securing, and utilizing data, potentially leading to DAQ Supremacy Technology, where data is not only abundant but also efficiently and securely utilized for a multitude of applications.
Big Data AI
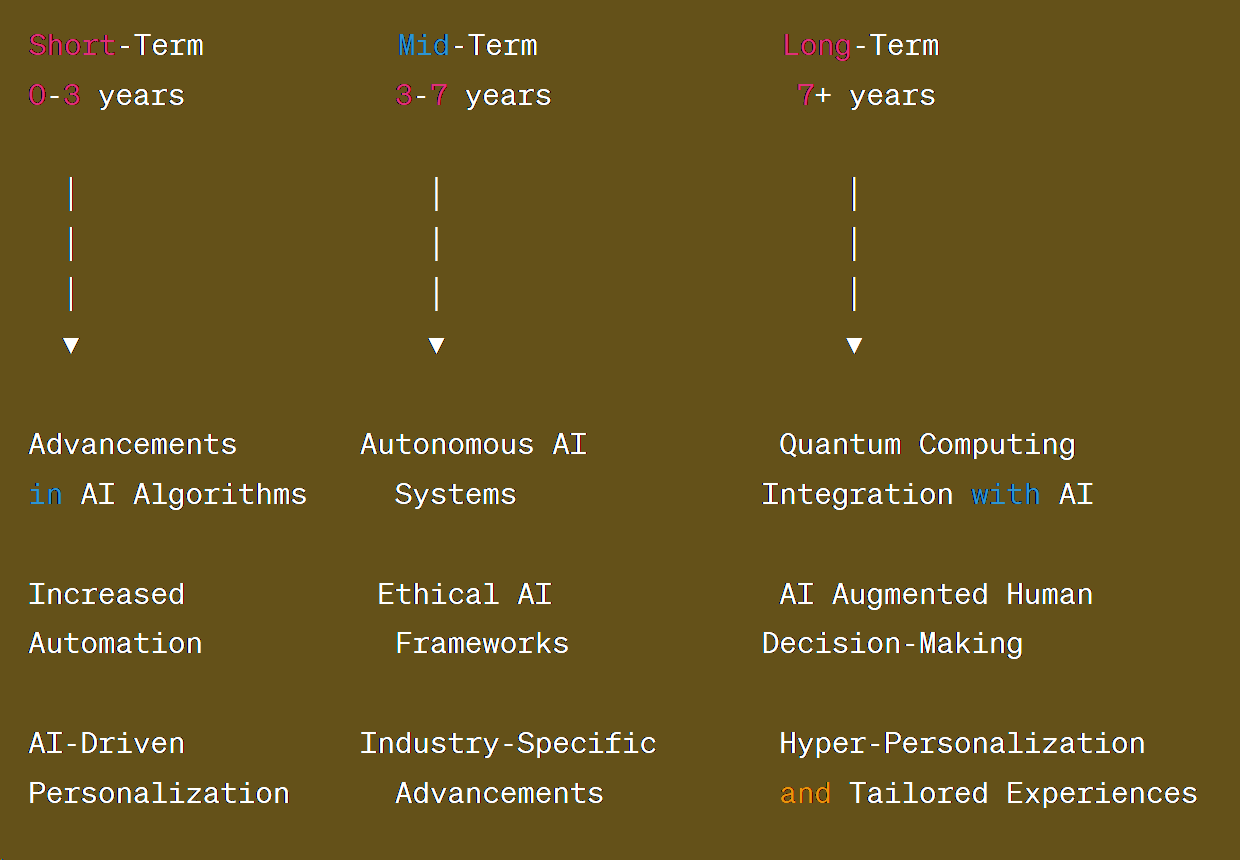
Simplified timeline structure showing the progression of developments in predictive analysis for DAQ across short-term, mid-term, and long-term periods. Each section highlights potential advancements and key focus areas in each time frame.
Trend & predictive analysis
Big Data and AI are revolutionizing predictive analysis across various industries. Here’s how these trends intersect:
Enhanced Decision Making: Big Data coupled with AI enables predictive analysis by processing vast amounts of structured and unstructured data. This analysis generates actionable insights, empowering businesses to make informed decisions.
Improved Accuracy: AI algorithms, particularly machine learning and deep learning models, enhance the accuracy of predictive analysis. They can identify intricate patterns within large datasets that humans might overlook, leading to more reliable predictions.
Personalization: Predictive analytics powered by Big Data and AI enables personalized recommendations and experiences in sectors like e-commerce, marketing, and entertainment. By analyzing user behavior and preferences, businesses can tailor offerings to individual customers.
Risk Management: Industries like finance, insurance, and healthcare leverage predictive analysis to assess risks. AI algorithms process historical data to forecast potential risks, fraud, or health issues, aiding in proactive risk mitigation strategies.
Supply Chain Optimization: Predictive analytics driven by Big Data and AI helps optimize supply chain operations. It forecasts demand, identifies potential disruptions, and suggests inventory management strategies, improving efficiency and reducing costs.
Healthcare and Precision Medicine: Predictive analytics in healthcare utilizes patient data to predict disease outbreaks, personalize treatment plans, and forecast patient outcomes. This aids in proactive care and development of personalized medicine.
Energy and Resource Management: Predictive analysis assists in optimizing energy usage and resource allocation. It forecasts energy demands, identifies inefficiencies, and suggests ways to optimize usage for sustainability.
Challenges: Challenges in predictive analytics include data quality, privacy concerns, biases in algorithms, and interpretability of AI models. Addressing these challenges is crucial for ensuring accurate predictions and ethical use of data.
Continuous Learning: AI-driven predictive models continuously learn from new data, refining predictions over time. This adaptability improves the accuracy and relevance of predictions in dynamic environments.
Real-time data solutions:
Predictive analysis with AI
Real-time data solutions combined with predictive analysis powered by AI represent a cutting-edge approach to harnessing data for immediate insights and future predictions. Here’s how this process generally works:
Real-time Data Collection: Utilizing various sensors, devices, or systems, data is continuously gathered and streamed in real-time. This could include information from IoT devices, social media, online platforms, sensors in machinery, financial markets, or any other relevant sources.
Data Processing and Integration: This incoming data is processed and integrated into a central system or platform. Real-time data processing technologies handle the streaming data, ensuring it’s cleaned, structured, and prepared for analysis.
AI-Powered Predictive Analysis: AI algorithms, such as machine learning and deep learning models, are employed to analyze this real-time data. These models learn patterns and relationships within the data, allowing them to make predictions and identify trends, anomalies, or potential outcomes.
Actionable Insights: The predictive analysis generates actionable insights in real-time. This could involve identifying potential risks, anomalies, opportunities, or trends that businesses or systems can act upon immediately.
Adaptive Learning: As more data is collected and analyzed, AI models continuously adapt and improve their predictions and insights, making them more accurate and valuable over time.
Benefits of Real-time Data Solutions with Predictive Analysis using AI:
-
Immediate Decision-making: Real-time insights enable swift decision-making, crucial in scenarios like financial trading, cybersecurity, or emergency response systems.
-
Risk Mitigation: Early identification of potential issues or anomalies allows for proactive measures to mitigate risks.
-
Enhanced Efficiency: AI-driven predictive analysis automates the process of deriving insights from data, reducing the need for manual analysis and significantly improving efficiency.
-
Customer Personalization: In sectors like e-commerce or marketing, real-time predictive analysis helps tailor customer experiences based on immediate behavior and preferences.
-
Operational Optimization: Industries like manufacturing or logistics can benefit from optimizing processes based on real-time insights, minimizing downtime or improving supply chain efficiency.
-
Improved Accuracy: AI’s ability to learn and adapt from real-time data enhances the accuracy of predictions and insights over time.
-
Innovation and Competitive Edge: Leveraging these technologies can lead to innovative solutions and give organizations a competitive advantage in their respective markets.
The integration of real-time data solutions with AI-driven predictive analysis holds vast potential across various sectors, revolutionizing how businesses operate, make decisions, and serve their customers in an increasingly data-centric world.
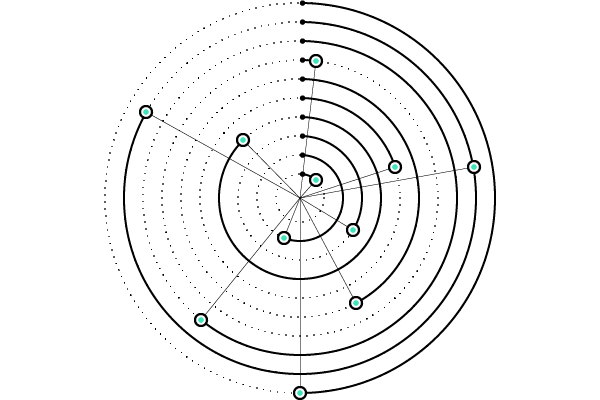
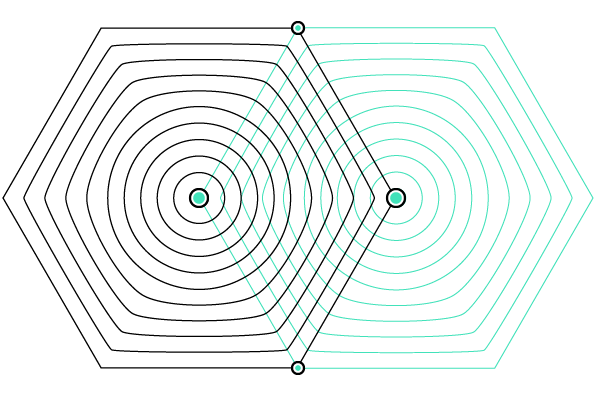
Pattern detection & analysis for predictive AI analysis solutions
Pattern detection and analysis play crucial roles in facilitating predictive analysis powered by AI. These components involve the recognition of regularities, anomalies, and trends within datasets to derive meaningful insights:
Pattern Detection:
- Recognition of Regularities: AI algorithms excel at identifying patterns within complex datasets, including correlations, associations, sequences, or recurring structures.
- Anomaly Detection: AI also has the capability to detect anomalies or outliers that significantly deviate from established patterns, vital for applications like fraud detection or fault identification.
Pattern Analysis:
- Feature Extraction: AI models extract relevant features from data to simplify complexity while retaining essential information, aiding in more effective pattern recognition.
- Temporal and Spatial Analysis: Analysis of time-based (temporal) or location-based (spatial) patterns helps uncover trends, crucial for fields like financial market predictions or temporal-based forecasting.
Predictive Analysis:
- Learning and Prediction: AI models learn from identified patterns and historical data to predict future outcomes, involving training on labeled data to understand relationships and apply this learning to make predictions.
- Forecasting: AI-powered predictive analysis forecasts future trends, behaviors, or events based on recognized patterns, influencing business planning, demand forecasting, or resource allocation.
Applications:
- Healthcare: Recognizing patterns in medical records aids in diagnosis, treatment planning, and predicting patient outcomes.
- Finance: Identifying patterns in market data aids in stock price prediction or fraud detection.
- Manufacturing: Understanding patterns in production processes optimizes efficiency and predicts maintenance needs.
- Customer Behavior: Recognizing patterns in customer behavior supports personalized marketing and product recommendations.
Continuous Improvement:
- AI-driven predictive analysis continually improves as more data is analyzed, patterns are refined, and models are updated, enhancing accuracy and relevance over time.